
Quantum Enhanced Optimization for Industrial-Scale Problems
William P. Banner | Tim Menke | Shima Bab Hadiashar | Grzegorz Mazur | Marcin Ziolkowski | Ken Kennedy | Jeffrey A. Grover | Jonathan Romero | William D. Oliver
Many of the most challenging optimization problems faced by industry today are combinatorial in nature. Quantum computing and related approaches offer new heuristics for tackling these problems that might provide advantages over traditional optimization methods. Establishing such advantages requires benchmarking on specific problem instances. In this work, we consider the production plant optimization problem under realistic conditions. We characterize the problem and carry out a benchmark of multiple classical and quantum-inspired optimizers, including techniques based on generative modeling for quantum enhanced optimization. By comparing classical optimizers, quantum-enhanced optimizers, and mixed optimizers that combine the two, we gain insights into which aspects of the problems influence the performance of the optimizers. In addition, we perform a scaling analysis of the optimization methods and estimate thresholds for advantage.
Funding Sources: MIT Center for Quantum Engineering
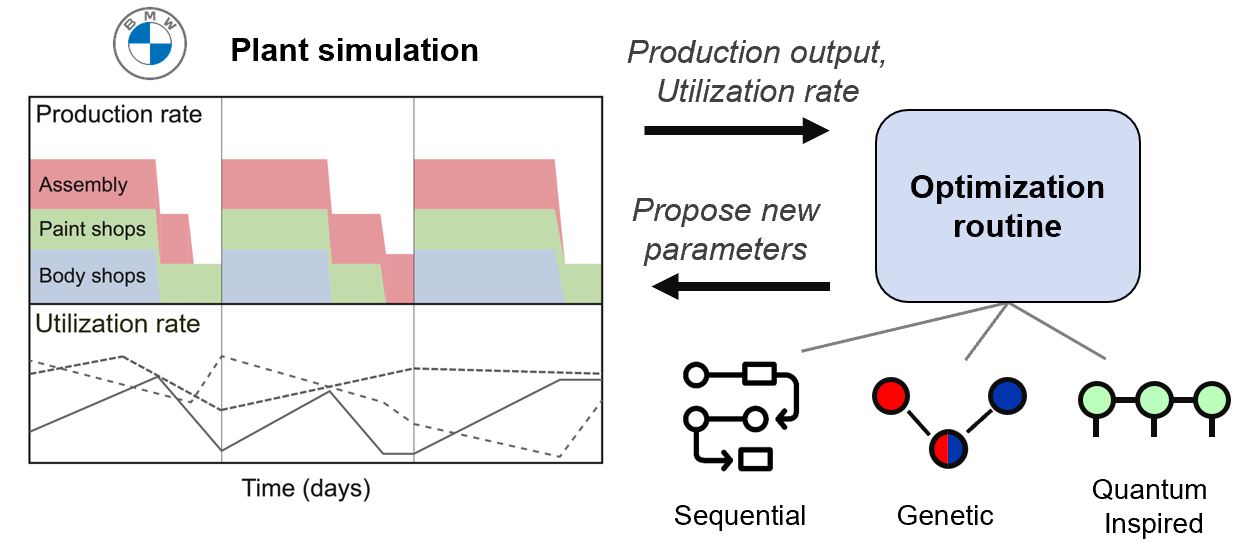
William Banner
Affiliation: MIT, Graduate Student
Areas of Research
-
- Quantum Algorithms & Machine Learning
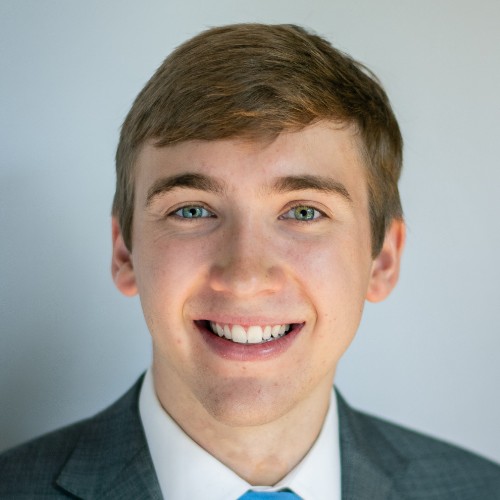